Empowering Sales Teams with Revenue Insights
Episode Description:
Today we sit down with Isaac Pohl-Zaretsky, the visionary CTO and Co-Founder of Pocus, to explore how AI is reshaping the B2B SaaS landscape. Isaac shares his incredible journey from building a massively popular gaming platform to founding a company that's at the forefront of the AI revolution. Tune in to learn how Pocus is leveraging AI to help businesses turn data into revenue, and discover Isaac's insights on the future of AI and entrepreneurship.
Resources Mentioned:
The Four Steps to the Epiphany
INSPIRED: How to Create Tech Products Customers Love
Superintelligence: Paths, Dangers, Strategies
Full Transcript:
Andrew Miller:
Welcome to another episode of AI Unboxed. We're going to go ahead and just jump into things today, and I am joined by Isaac Polozarecki, who is the CTO and co-founder of Pocus.
Pocus positions itself as the mission control for your pipeline.
It brings together product usage and other intense signals, such as customer, community, and marketing data, to surface top leads and enable sales reps to act fast.
Prior to launching Pocus in 2021, Isaac built and launched a massively popular role-playing game.
I'm going to jump into that in a little bit more, but when I say massive, I mean zero to over 5 million unique users.
And he built this within Microsoft's Minecraft.
Isaac, so glad to have you here on the show. Appreciate you joining us.
Isaac Pohl-Zaretsky:
Very happy to be here, Andrew. Really appreciate you having me on.
Andrew Miller:
Now, I know I gave a brief introduction, but is there anything else that you might want to like double down on or give the audience a little bit more insight about your background?
Isaac Pohl-Zaretsky:
No, overall, all good. I'll mention I have some experience doing AI and data work.
I spent some time at Airbnb working on machine learning infrastructure where I got a lot of exposure to various big data and AI challenges, which is great. Right.
Andrew Miller:
That's awesome. That's awesome. Well, I do want to look a little bit further into WinCraft. You know, what took you from the gaming world into B2B SaaS?
Isaac Pohl-Zaretsky:
You know, it's a it's a less common transition that you than you might think.
But honestly, at the end of the day, when I started Wingcraft and ran it for like five or six years, I just fell in love with creating solutions that helped a ton of people solve their problems.
Like, I can't tell you how fun it was as I was running Wingcraft to have an idea and ship it within a couple of days and then see like actually millions of people get to love it and get feedback and and start using the thing that i had built so it really gave me this bug of building things from scratch and as i went and worked in more traditional like tech jobs um i struggled not feeling that same level of like impact and like exposure to the impact of the work that i was doing so it made me really want to start another company um going from minecraft to b2b be SaaS was honestly a little bit of a coincidence of how it ended up.
I didn't set out to start another video game. I didn't set out to start a B2B SaaS business. My goal truly was just to build something else that people were going to really enjoy.
And as I started that process of learning about pain points and what possibly I could spend my time doing that feels like it would have a really high impact.
The idea for Pocus sort of came out as something that felt really clear and had a really desperate need. So that's how I ended up here. There's no master plan.
Andrew Miller:
Nice, nice. Well, I know there's a lot of prominent individuals inside of the B2B, you know, SaaS space.
They might not talk about it too much, but they, you know, hint towards gaming impacting a lot of their lives and drawing them into this world and business and entrepreneurship.
I know I'm a big fan of like Eric Hsu, and he, you know, created an entire community that uses gamification and talks about all up-leveling and leveling up.
I think his book was called Leveling Up.
And it has a lot of those gaming aspects built into there as you achieve new unlocks as you go through there. So very, very, very, very cool, man.
I know that AI Unboxed is really focused on AI.
So what aspect or what fascinates you about AI and how are you kind of bringing that into Pocus?
Isaac Pohl-Zaretsky:
Yeah, a ton. Honestly, I think the way that salespeople and go-to-market people have been working over the last 10, 15 years has stopped working.
And it's not because AI was invented that it stopped working.
I think it's a cause of the recession and a lot of companies having a much smaller sales team than they used to.
It's because of a lot of changes to how buyers want to be sold to, right?
Like steak dinners and getting cold calls isn't the way that people want to be sold to anymore, right? People want to think about products that they're using and talk to people that they actually want to get information from.
So we've seen that trend of product-led growth, and a lot of the teams that we work with have seen incredible success with product-led growth, where instead of cold calling, they're able to build incredible revenue engines just by having people love their product.
A lot of what we're thinking about is how do we make it so that every interaction between a buyer and a seller is that same type of interaction, where the buyer wants to be talking to the seller and the seller isn't bothering the buyer. Right.
One of the things that really excites me about AI, and I think when I think about go to market and sales and customer success and the intersection of AI is it can really change the way that people are interacting with their with their buyers.
If you think about how a customer success or a salesperson goes out into the market and talks with their prospects, the fact that they can use data to actually bring exactly the right pain point to the buyer and use AI to bring exactly the right messaging and really identify exactly the right things in the phone call or in the email totally changes the way that relationship works.
So a big thing that we're thinking about when we think about AI and go-to-market is how do we leverage AI and other types of data to make it easier for sellers and customer success folks to bring exactly the right message and tone to every conversation? Yeah.
Andrew Miller:
Yeah, yeah, absolutely. And that, I guess, that creates that one-to-one personalization that everybody is really looking for.
And everybody has been shouting from the rooftops for a long time saying, hey, we're able to bring you this one-to-one conversation, give you all the insights, but it hasn't ever actually been there, right?
But we've made so much progress in just the last few years, especially with, you know, big data, machine learning, being able to really give you those insights that we've always been like looking for.
So that's amazing. I know you mentioned product-led growth, but one thing that, and I mentioned this when I was talking to Sophie, is Pocus, in my perspective, and the first time I ever got introduced to it, was a term called product-led sales.
Could you maybe elaborate a little bit more about what that is and how you're leveraging that?
Isaac Pohl-Zaretsky:
I'd be very happy to, yeah. So the day we started Pocus, we had the same vision and mission that we do right now, which is we wanna help our prospects, We want to help our customers turn data into revenue.
That is what Pocas is. We are a company. We are a platform that helps our customers turn their data into revenue.
We started really by staying very, very focused on a problem that we call product-led sales.
And that problem was, how do we help companies that have really strong product-led growth motions build sales and customer success motions on top of that?
So we built a really powerful platform. It's still the platform that powers everything that Pocas does today, that allows customers to bring data together from their CRM, from their data warehouse, from tools like Segment and Snowflake, all into one interface to make sure that they are spending time with the leads that want to have time spent with them.
And they're bringing the right messaging to every conversation, right?
So we work with teams like Canva and Asana and Monday.com and Miro and Loom, where their sales teams are using Pocas in order to decide where should they be spending time.
Their CS teams are using Pocas to decide where are the best expansion opportunities And what customers do we need to be working with to prevent a churn?
So that's kind of how we started. As we've gone down this journey, what we found is there's all types of data that can really help people do their jobs better, right?
Part, like we started with product data as the main signal in POCUS.
But every single request that we get is, hey, how can I find out if they talked about product management on a block, right?
We hear requests like, hey, did the founder in a podcast talk about productivity or communication in the last quarter, right? I did their 10K mention how they can leverage AI to make their productivity higher.
So one thing that we've been thinking a lot about and a lot of where our direction has been over the last few months and going into the next year is how do we bring other types of data to help all types of teams, not just product growth teams?
Andrew Miller:
Very interesting. Very, very, very, very cool. Yeah.
Now, if we if we take a moment and look at the industry transforming in the future, right, so taking a step back from what we're talking about, but looking at today, and then in the future, how is AI revolutionizing in your perspective, the B2B SaaS space today?
And could you share maybe a real world example?
Isaac Pohl-Zaretsky:
Yeah, absolutely. So the way that we think about it at POCUS is there's really two ways that AI can have a really meaningful impact on how B2B SaaS and specifically go to market teams within and B2B SaaS companies operate.
One is AI is making it easy for people to have a lot of different types of data than they had in the past, right?
Traditional sellers where they're working just out of a sales profile are really reliant on firmographic data, right?
Like job title, maybe activity data of what marketing materials have they interacted with or what emails have we sent them.
But with AI, we can pull so much more data about who the person that you're selling to is, what pains that company is experiencing, financing, what they're talking about on social media, what they're talking about in their 10ks, what they're talking about in past emails from two years ago with you, right?
So I think that's one huge category of how can we bring better data to reps so that they can have more thoughtful, intelligent conversations with everyone that they're working with, instead of having kind of the same conversation based on a firm of graphic data, which is really limited.
So that's one big category is more data and lots of stuff that we're working on really specifically in that that I'm excited to chat about.
The other category that we think about is like, Like, how do we make it easier for people with NB2B SaaS companies to do their work, right?
Like, put simply, how do we save them time and make it so that one person can do the work of what 10 people could do a few years ago?
And there's a lot of ways that AI is helping us with that. You know, really basic features that we've already put in our platform.
Stuff like email writing, right?
Automatically writing the hook to an email so you can write mass personalized email sequences is really important, right?
Stuff like automatic prospecting and prioritization of prospects.
Aspects so that when you were looking at your dashboard and Salesforce or in Pocas, you're not seeing a list of 10,000 leads.
You're seeing a list of eight leads that you should actually spend time with today. And you don't need to sift through all the rest.
Stuff like that really drastically changes what the job is for a seller, right?
Instead of that person being there to scrape through data and go through 10,000 people and find those eight people, they really get to narrow down on what's the most important high opportunities that we have.
And what's the fastest way for me to go and action these people.
It really is like a lever on top of what people can do.
Andrew Miller:
Very nice. Very nice. So would you say that you're incorporating some sort of like lead scoring functionality into that?
Is that how you're like saying these are like the top customers that that people should go after?
Isaac Pohl-Zaretsky:
Yeah, we have all types of different ways. Like broadly, we think about this problem as how do we prioritize opportunities, leads, accounts within Pocus.
We have a lead scoring system in Pocus that's really powerful.
One of our products is called Pocus Predicts, where we actually look at all of your historical conversions.
So we say, what does someone who is a close to an opportunity look like?
Or what type of people are responding to emails or booking meetings?
And we can find trends within the data of those people. So for a product like Growth Company, it might be, hey, the person who's responding to your email, it's the same person who's inviting their co-workers to the workspace right or it's the same person who's been downloading your webinar or it's the same person who on linkedin talked about ai productivity in the last couple months so we can help you find those patterns and then identify the top people um we have lead scoring we also have a feature in focus called playbooks where we can actually have different categories of why people are being serviced to the reps um again making it really easy for sales cs people to action only the data they need to to be thinking about and not being inundated with like hundreds of millions of rows.
Andrew Miller:
Yeah, yeah, no, that's so helpful. I mean, you touched on a lot of really great things there.
I mean, basically, you're mapping out true customer digital, I mean, digital body language, right?
And you're not isolating yourself just inside of the product, but you're going outside and actually doing like social scraping and pulling in those data points, and then using AI machine learning to map out all these different touch points and say, these are the highest probability people people based off of the data that we're seeing in the interactions and everything around there, which is truly like the holy grail that people are always looking for, because I've used, you know, so many tools in like my 15 plus years in marketing where, oh, you can just see this little segment, maybe it's just product data.
And so you just get that insight.
And if you want to go outside and do scraping, you have to use another tool.
But then that tool is limited to only a couple platforms. And it's like, oh, what about over there?
And then you try to pull that in and then you pull everything in there and manually like build a spreadsheet to pull this data together and it's yeah it takes up way too much time and it is not even 100 accurate because they have different uh attribution models and different you know things so yeah yeah no i love how you've housed everything inside there uh and and utilizing the tools that are now becoming more you know apparent building the tools you know that that you need um, Speaking of the future and already the progress that's happened here, how do you anticipate AI will actually transform, you know, let's go, you know, a few years ahead, transform the B2B space?
Isaac Pohl-Zaretsky:
Yeah. So I think the two trends that I was talking about of really different data that reps are using to do their jobs and reps having a very different job will continue.
Right. And I think when you think about the job of a CSM, when you think about the job of an AE or an SDR, five, 10 years from now, I really feel confident that's going to be a really different job than what it was five years ago.
Right it's going to be those people are going to be data workers right where they are consuming data deciding how to action that data and then actioning in the most appropriate way rather than i think what sales look like your cs looked like a few years ago where it really is like you're more of an operator on top of you know how customers work and and their data and all that so i think that's one huge thing it's like i think those two trends will continue i think the other big thing that we're going to start seeing more and more of and it's something that we're thinking a lot about and focused right now is experimentation and really taking a experimental mindset toward how you run go-ahead teams.
Historically, a lot of the ways that teams would decide how they're going to spend time was a little bit gut-based, right?
It'd be like, all right, this week, we're going to do a closed loss play and we're going to talk to every customer that we lost last year, right? And then this week, we're going to talk to everyone who signed up for a webinar.
And then it's sort of a series of activities and actions that are assigned to people that aren't necessarily tied to actual results.
So something that we're thinking a lot about is how can we help our customers run experiments using AI to decide what the experiment should be, but then also have A-B testing and actually run go-to-market teams in an experimental way, where you can have really confident results of, hey, this is having a real impact.
It's not just that these people are likely to convert, but our salesperson sending an email with this cadence at this time is actually having the impact that we think it's having, so let's keep doing it.
Andrew Miller:
So what would you say are some of the challenges in bringing POCUS, you know, to life, but also, you know, some of these newer like POCUS Predict and AI models and everything incorporating those into it?
Isaac Pohl-Zaretsky:
Yeah. So what you brought up earlier, Andrew, of there's all these different tools to decide, you know, we're going to scrape this data from here and we're going to pull this list from here and then it'll end up in a Google Sheet and that Google Sheet will end up somewhere else. else.
The root cause that I hear when I hear you talk about those issues is there isn't a tool that's flexible enough to actually capture what you're trying to do.
What we found, and we felt this really acutely when we started with product data, and I think it's a real gift for us as a business that we started with that type of data, is every company just has a really different shape of what they are looking for in a customer.
They have a really different shape of what their data looks like within their systems.
They have a different set of tools that they're using and different types of insights that they're trying to bring together.
So one of the really foundational choices we made with Pocas is instead of trying to ask all of our customers to fit into this like Pocas model or Pocas schema of how things should work, we're going to give you the tools to build your own model, your own schema.
So that shows up in a lot of ways in our product, right? Like you can have unlimited objects in Pocas and unlimited types of data per object, right?
You can have unlimited scores in Pocas and multiple experiments and playbooks running for each different type of object.
And it's really cool because when you log into one customer's workspace who's working on, on, I don't know, a productivity tool versus another customer who's working on a sharing tool or some sort of content creation tool, their workspaces in Pocas look drastically different, right?
Like the way that they're interacting with data, the way that they're servicing data, it is significantly different between one customer to the other.
And it's a really hard challenge as a creator of this platform, right?
Of like, okay, I need to think about how Asana's sales motion can fit into the same product that's going to support Canva's sales motion, that's going to support Loom's sales motion.
Like there's all of these different constraints and goals that folks have.
I think that it's both a challenge and a fascinating problem that we get to work with so many different types of teams with so much different type of data.
As I think about the challenges going forward and what makes this a really interesting and fun problem is what we are tasked with is making every company have the perfect experience with Epocus, right?
We want them to have exactly the data and exactly the playbooks and exactly the models that are relevant to them.
But we need to do it in a way that works across all of them.
And it's like an exciting challenge, especially when we bring in AI of how do we predict what's going to happen next for 15 or 20 or 30 totally different types of businesses at the same time.
Andrew Miller:
Yeah, yeah, absolutely. So do you lean heavy on kind of like a product led motion yourselves inside of Pocus and that people can self serve and they go through there and then they have all of this like walkthrough onboarding.
So they're like, OK, you have all this that you can customize.
Here's how you do it. And you kind of walk, you know, go through it.
Or do you lean more on like the enterprise side where you have, you know, white glove onboarding and it's like, you know, Asana is very different than Canva.
How do we set it up? We need to do a consultative approach there to figure out what is your end goal and then build it out there for them.
Isaac Pohl-Zaretsky:
Totally. So everything in Pocus is totally self-serve. Like there's no custom things that we're doing for one of our customers over the other.
That said, and this is really embarrassing given how much we've been pounding the table about product-led growth the last couple of years, we don't have a product-led growth motion right now.
It's something that we want to do and it's something that we will be doing in the next, couple months or years or whatever. But what we found is, especially when working with product data, there's just enough sensitivity around security, and there's enough complexity in the setup that it's not worth us trying to get customers to do that without talking to us.
We've talked with folks that are solving similar problems who've just not seen success with that version.
So as we think about other types of tools and functionality that we're building in Pocas, absolutely, we're really excited to make it totally self-serve.
And folks can onboard word without talking to us. But for now, with our core platform, it is something you need to go through a more traditional sales process to get to.
Andrew Miller:
Yeah, yeah, makes a lot of sense. And you touched on something that's really important and leads into my next question. It's about the ethical considerations, right?
Especially whenever you're working with large companies. I mean, any company nowadays, you're handling data privacy.
You have to go through InfoSec, you go through SOC2 compliance, you go through GDP, all of those things that are thrown on there to make sure that the data is protected.
And now they even have like riders that are built out specifically for utilizing AI inside there and trying to dig into like, what are the LLM models or whatever else that you're using in there to aggregate the data?
Where is that open source? Is it not? What ethical considerations would you say are essential in your AI work?
Isaac Pohl-Zaretsky:
Yeah, absolutely. So I think the first big one for us is transparency.
Like so many companies that we work with and talk to and hear about are selling AI models like as a black box, right? If If you ask them, hey, how does it work? The answer is it's proprietary.
And our goal is that that is never the answer for anything that happens in Pocus.
Like even as we talk about bringing new data into the fold and helping our customers bring different types of data into their systems, we are ruthlessly transparent around where it's coming from.
Like we'll tell you the vendor we got it from. We'll tell you who we're integrating with.
Often it's actually not us integrating, it's our customer. Like we really, when we are working with data, when we're working with AI, we don't want it to be a situation where it's like the Pocus black box told you this is true.
Wanted to be a situation where we are the tool that our customers are using to get access to the stuff they.
So that's one really important principle to us is we want to be really upfront with where everything came from, how it's being used, where it's going.
There's no magic. There's no black box around how we handle and process data.
The other thing is being really thoughtful around. And last thing I'll say on transparency is we want to be really upfront with how the models, how the AI is working under the hood.
So something that you'll see within Pocas is whenever we give you a score, whenever there's a lead score, an account score, we actually show you all of the math of how we got to that score.
So we get some interesting explainable AI techniques to say, hey, this account is good or this account is excellent because X, Y, and Z works this way.
So really trying to make sure, never trying to assert something.
We're always trying to share this experimental process with our customer.
The other thing that we spend a lot of time thinking about is how is our product being used and what impact does it have on the broader ecosystem that we work in?
Right so we can have the best data privacy practices and the best ai transparency practices in the world but still build a product that's like causing people to be spammed or still build build a product that's you know causing people to have results that they don't want so one of our really important goals with pocus is we want every cut every person who interacts with our product or the output of our product to be really happy with that result right so for teams that we work with for customer success marketing sales teams what that means is we want this to be a tool that that they love and are excited to use.
And really importantly, as we talk about ethical considerations, when we think about customers that are being contacted because of insights from Hocus, we want them to be excited about that insight and not bothered by it, right?
So our goal is truly to stop spam and to make every email feel relevant and exciting as you're receiving it from a company, instead of it feeling like you're getting this bulk outreach that's going to another thousand people.
We have a lot that we're working on to make that happen. A lot of our functionality and platforms are already built towards that.
But that's the broad goal, It's like we want people who are getting contacted because of POCUS to be thrilled by that contact, not to be bothered by it.
Andrew Miller:
Absolutely. No, no, I love that. And I think you hit on a lot of really important parts that I think our audience is very keen to understand.
It's transparency is number one. Whenever people ask you, you know, what's behind the hood, you don't have to go into all the granular detail, but you can share, you know, a little bit of information there and show where these third party sources are and where the data is being tracked.
I mean, that's just like good business practice. practice.
Something unique that you just talked about is actually explaining how the model works whenever you're saying this is our predictive scoring.
That's really, really unique. I know it's not an ethical consideration, but it's really unique to me because if people are looking at it and they're like, oh, where did this come from? Why are these people being ranked as the highest?
Here's the model. Oh, if we tweak this one in here, maybe this should be the input as opposed to that. That'll give us our desired output.
And that can give you better data sets.
Isaac Pohl-Zaretsky:
Exactly. And honestly, like one thing that we talk about all the time with our customers, with prospects is all of this prioritization work, whether it be building a playbook or building a score or building an account list that our reps can be working out of.
It is an art and a science, right? There is a science element to it.
And HocusPredix gets at that really effectively, right? We can help you identify what are the trends historically that have been happening.
But there's a real significant art to it as well, right?
Like there are virtually zero customers who say, we want to repeat the year we had last year, next year, right? Virtually everyone that we work with has some new strategy or some new goal or some change they want to make in their business that's not going to be reflected in the historical data that we're able to train models off of.
So within Pocus, when we're defining scores, it's not a black box.
There's an AI model that will create weights and suggestions for the data points that will go into the score.
But humans can actually take all those different levers and tweak them around and say, actually, we want to go a little more upmarket this year.
It hasn't been our persona in the past, but we're going to try it out.
And they can literally just push a lever for larger companies get higher rating, and they'll They'll actually see the change in real time to how that impacts their prioritization.
So we're totally on board with that as well. There's a huge element of it of how can we use science and experiments to get to the bottom of some of these answers.
But there's also a human element as well that sort of needs to coexist.
Andrew Miller:
Yeah, yeah. In your journey to incorporate AI, because I know it hasn't been publicly available to everybody.
I mean, AI has been around for a long time. Our founder at Zimbly, he founded MarchX, which was a conversational AI company, what, what, like 10, 15 years ago and brought it from nothing to IPO'd.
And so he'd been working in that space for quite a while.
But every company faces some sort of challenge or dilemma as they're bringing it to market.
Was there a specific ethical dilemma that you're open to sharing that maybe you faced in incorporating it?
Isaac Pohl-Zaretsky:
Yeah, I was honestly, I was rocking my brain earlier. I mean, like I think broadly, transparency and quality that's being used is important.
But like the reality at the end of the day is like, emails getting sent or not getting sent, it's not the most ethically important work in the world.
I actually, I'm fascinated by AI fairness and AI ethics. I spent a lot of time reading articles thinking about it.
And I think the things that matter to our criminal justice system and government, it's not as much like how salespeople are reaching out to folks.
I think the principles that we lean on are really important to be like well defined, so that as we make good decisions, they continue and we aren't, you know, threatened or suggested by some like different idea to do something that doesn't feel ethically responsible to us.
But we've been fortunate that there haven't been a ton of like specific occurrences that have popped up for us just yet.
Andrew Miller:
Awesome. Awesome. Well, I mean, that's similar to like Process Street.
I had Vinay, the CEO on here a while back, and I asked him that same question, you know, have you faced any ethical dilemmas there? And he's like, no, no, not really.
I mean, we're not getting any like internal data from customers, I mean, from our customers about their customers.
And we also have this toggle switch, what I'd never heard of, because they built their product before incorporating AI, which a lot of companies have done.
And they actually built a function inside there that lets you toggle on the ability to incorporate AI into the workflow processes and everything and toggle it off. And I'm like, well, that's smart.
If they do have any concerns, they just turn it off and there you go. Yeah.
Isaac Pohl-Zaretsky:
We actually have the exact same thing. Some of our larger enterprise customers, they're just not comfortable with it getting used. And that is totally okay.
There's a lot of value you can get out of Poco without using AI.
Andrew Miller:
Absolutely. If we look into the future a little bit, what What would you say is a groundbreaking, well, I guess it's not future, it's current, but a groundbreaking yet underutilized AI technology in the B2B space?
Isaac Pohl-Zaretsky:
Yeah. I want the answer to be generative AI.
And I actually still think it's massively underutilized, but it's something a lot of people are talking about, right? I think a lot of LinkedIn and folks online are thinking a lot about it.
My hot take is like, I think there's also an enormous amount of unique ground to still be covered with more classical machine learning models, non-generative AI. They're just use cases where it makes sense to use that.
So we have a lot of places where we've gotten to implement generative AI at POCUS, and it's been awesome.
But things like, hey, over the last year, what are the common data points that make all of our 15,000 open opportunities in common?
That's not an easy question to answer with a generative AI model.
In fact, I don't think it's a possible question to answer with AI models.
And one thing that we found is when customers Customers are saying AI, they're not saying generative AI, right?
What they're saying is like, I want this product to tell me what to do instead of me telling a product what to do, right?
And I think that there are different tools for different jobs.
And we found a lot of success using generative AI models for a lot of jobs.
But I think a lot of companies are like really trying to buy into this hype cycle and like release their generative products, but still have a lot of places where their products are not doing the right thing, where they're still asking their customer, what do you want to do instead of telling their customer what they should be doing?
And I think there's a lot of opportunity for folks to rewind one year and make sure that they're adopting all of the types of tools that exist for AI, not just the new generative stuff.
Andrew Miller:
Yeah, yeah, absolutely. I think that the holy grail that people are looking for is something to do the work for you, you know, not just like give you the output and like, here, figure it out.
So I'm not going to go on the side about Zimbly and everything about us, but you know, check it out.
Where do you think, and you talked about it a bit already, but if you want to double down, where does AI fall short in this space?
And how can they overcome those limitations? I think that's more important. Yeah.
Isaac Pohl-Zaretsky:
I will answer it in two ways.
One, I think technically, is I think there was a lot of hype initially right when AI came out, or generative AI started becoming popular, of we can replace all emails with AI.
And we have a product in our, we have a feature in our product that writes hooks, right?
It's, hey, the one sentence, hey, Isaac, see you also went to Stanford, you know, go Cardinal, let's talk product, right? That I actually think is really effective.
But I've seen a lot of LinkedIn posts and people trying to start companies where it's like, we're going to write your entire email and your entire strategy based on generative AI.
And I'm not convinced if it's just impossible or if generative AI just isn't there yet.
But I've yet to find a success story where folks have gotten entire sequences and outreach strategies built just using AI.
Who knows how that will develop? I would love for the answer to be that there will be developments in the future, are technically or genius product developments that will make that really different.
But so far, what we've found is AI is really helpful for the personal aspect of an email, but it's not important for, hey, how do you describe the value prop of Pocus to a customer?
That's kind of similar whether or not generative AI exists.
That's one area. I think the other area of where AI is still falling a little bit short within sales and sales tech and CS tech is really making things accessible to end users.
I think that there are a lot of companies that are using AI in a way that's really focused for back of house operations people, right? Like I think lead scores are a great example of that.
A lot of teams that we work with have these really sophisticated lead scoring algorithms that are these like monstrous black box AI models that are actually really good. Like I think their accuracy are really, really solid.
But then I talk with salespeople who are supposed to be using those lead scores and they just say that they ignore them, right?
And the reason they ignore them is they see in Salesforce, they see this lead had a 98% chance of responding to their email, and they didn't respond to an email.
And the salesperson says, what? This 98 meant nothing, right?
And they go through that list, and that happens a few times a day.
And very, very quickly, that number starts getting north. And instead, they use their own intuition around what they should be doing.
So I think that even though we have these amazing tools to prioritize things, I think that there's a lot of shortcomings from how people have implemented it, where they're just not doing it in a human way.
That's making it really hard for sales, customer success, like I see is to actually make a difference in their day based on the data.
And that's something that we're thinking a lot about at POCUS, of how can we bring these amazing insights, new types of data or AI models into a workflow in a way that feels really seamless and helpful instead of feeling disruptive and something that is very easy to lose trust in.
Andrew Miller:
That immediately made me think of multiple examples in my past, you know, where the data science team just loved going in there and building out these, you know, predictive score models in the back end.
And they're super complicated and they use a lot of data sets in there.
And the sales team starts looking at it as like, OK, so they should respond. They didn't respond.
You know, what happened here? Let me go. Let me go do another one.
Let me try that. It's not responding. Never mind. This is like not useful for me, you know, at all. all.
It also makes you think of like Dan Ariely and his book, Predictably Irrational, right?
As humans, we can look at the larger data set of people and what we anticipate that they should be doing from a logical standpoint.
But a lot of us just aren't logical. And there's additional factors outside our current control that maybe they got busy.
Maybe they just had a baby. Maybe something else happened.
And it's just, even though the model says they're going to respond, they don't respond.
So it's like you mentioned earlier, you know, a blend of art and science whenever you know, we're working on this.
So yeah, something that makes it challenging and fun, right?
Isaac Pohl-Zaretsky:
Absolutely. Yeah. And some of the stuff that we try to do in POCUS is really lead with what happened instead of leading with our score, right?
Like the reason a person's a 98.
It's not because of math. It's like this person downloaded the webinar, right?
Or it's because this person signed up for the product invited three people and has been active since then, right? Like that's That's what's important.
So we try to make that the headline. It's like when a rep sees an alert from Pocus, they're never seeing, hey, this is an 85%.
They're seeing, you just had a director at the company who looked at your pricing page for eight minutes, then they downloaded a webinar.
That's a really good sign, right? Like that's the information they're getting from Pocus, which we find works a lot better because it's more trustworthy.
It's not something that they're gonna like stop trusting the next time.
Andrew Miller:
Yeah, yeah, absolutely. That gives them the why and the behind the scenes, like, oh, okay, that makes sense.
Let me follow up with them, as opposed to just a score, like you said, like a black box and they have no idea.
Isaac Pohl-Zaretsky:
Exactly, yeah.
Andrew Miller:
Now we can get into the future outlook. You know, what emerging AI trends would you say that you're most excited about?
Isaac Pohl-Zaretsky:
Yeah, that's a great question. I think the continued intelligence from generative AI is really exciting.
I think that one of the things that is still challenging about use of generative AI is it works 90% of the time and not 100% of the time.
And that makes it really hard to automate it, right? It makes it hard to say, hey, send an email to every one of my target customers because maybe 10% of those emails are going to look right And it's going to be a wasted opportunity.
So that's one thing I'm excited about. I think as these models develop and get better, it's going to be a lot easier to actually trust them and ensure that they're going to do the right thing 100% of the time, which is really exciting on the generative AI side.
I'll also say one thing that I'm really excited about is the progression of how AI is going to impact experimentation.
One thing that I've been reading a lot about, and it's been honestly driving a lot of how we're thinking about the long-term technical vision for POCUS, is real-time interactive experimentation.
And there's some really cool stuff that's happening. I think Netflix has a really good research paper about this.
Google does this with ads, but it hasn't translated to the B2B world yet of automatically trying something new, trying with six people.
If those six people respond well, expand it to 30 people. If those 30 people respond well, expand it to 1,000 people and kind of have that be the way that teams drive strategy of how they're going to do outreach or how they're going to sell their product or how their vision for the product is going to be articulated, right?
I think that those automatic real-time experiments where there's an AI generating the experiment, there's an AI rating the experiment, there's an AI facilitating how many people are getting treatment A versus treatment B versus treatment C is really, really exciting. And I don't think anyone's realized that vision for B2B yet.
Andrew Miller:
Wow. Wow. No, no, I love that. I mean, with my background, I primarily come from the growth space.
Right. And so you're always running different experiments, but you have to try to get some sort of statistical significance in your data point.
And if you don't have enough people or enough time, you usually don't get there.
And it's like, well, that didn't hit significance.
Let's let's try something else. So let's do another, you know, another version of this.
Um, so having something that can look at this like micro scale, uh, in real time and then just build onto it based off of interactions, uh, yeah, that, that opens up quite, quite a lot. Uh, yeah.
Sign me up, sign me up right now.
Isaac Pohl-Zaretsky:
You know, we'll, we'll shoot you an email when it's ready. Yeah.
Andrew Miller:
Yeah. Um, how do you see the relationship between AI and the human expertise, you know, evolving over time?
Isaac Pohl-Zaretsky:
Yeah, that's a great question. um i think that what is really exciting like when chat gpt dropped a year ago right and i think everyone was oh my gosh human knowledge work is over and we're gonna be replaced just like how you know cars replace horses i don't think that is what's happening right and like maybe i'm gonna watch this podcast back in 25 years as my like ai robot overlord is telling me what to do i'm gonna feel differently but i think what's really exciting is that's not what's happened i think think what's happened, and what I expect to continue to happen, is AI will act as a really significant lever for human expertise, instead of humans having to do their own route boring work, right.
And I think that's already what we're seeing with our customers, right, where we are genuinely able to take every AE in SDR, and give them a force multiplier by giving them an army with data and insights that are really important for them to do their jobs.
And I think that as AI gets better, that force multiplier only goes up, right, where instead of needing a 40 person team to do something, you need a six person team to do something.
That's what I think is really, really exciting about this is when AI gets realized to the fullest extreme and we're able to type in the name of a product and it exists overnight.
It's amazing because instead of the work of creating a startup, doing the work that we're doing, instead of us hiring a bunch of engineers and spending a lot of time coding and thinking about that, that doesn't need to be my day if Generative AI fully exists. this, right?
Like each individual can be working and finding the best solutions to a problem, like the true human problem of what do we want to do instead of the actual like tactical work of, you know, building a team, writing code, whatever.
All of that's fun. Like I don't think any of it's going to go away entirely.
But I think that AI is going to let us have one person do 10 people's jobs, which is which is like a really, really exciting future to think about.
Andrew Miller:
Yeah, yeah. It's allowing us to really focus on the meaningful, right?
Focus on the things that that are moving us forward as a, well, on a business, on a business standpoint, but also as like a civilization, you know, instead of doing a lot of these mundane tasks, which was the entire point of technology, when you really think about it, right?
Technology was built to help us evolve and focus on more strategic and higher level function.
But what's happened is a lot of times we get pulled into the very granular detail that shouldn't, doesn't really move the needle, right? Right.
It's like you're sitting there and like an example, like taking meeting notes.
Is that really a best use case of a person in a call sitting there and just taking the notes and not being able to interact?
Because when you're taking notes, you can't think about what's going on and, you know, actively contribute to anything.
So I love that explanation and I'm excited for that as well.
If we look a little bit towards like your personal insights and lessons throughout your career, what lessons would you say you've learned? it just in your career that you wish to you know share uh maybe that you knew earlier you know.
Isaac Pohl-Zaretsky:
Yeah. All right. I'll give one answer not AI related, and then I'll give an AI answer.
I think the non-AI answer is really driving behavior based on actual conversations with people.
I think that a lot of people in my shoes who come from a more technical background build a lot of product and a lot of technology without talking to anyone, and it doesn't work.
And I think that 100% of the success that we found with Pocus, it's not because I'm a great entrepreneur. It's not because Alexa, my co-founder, is a great entrepreneur.
It's not because our team is an amazing team, even though I think our team is amazing. And I think Alexa is a great entrepreneur.
I think the reason that we've been really successful is we've been lucky enough to get to spend time with really thoughtful customers and really, really thoughtful experts in the space.
And we just listen to them and build the things they're asking for right like there's no genius epiphany that happened overnight like we didn't wake up from a dream and have the ideas to do what we've been doing i think we just built a really amazing practice of like building close relationships with smart people who are thinking hard about things and then helping them build the things they're looking for um so that's one big lesson that i think has been amazing for me as i as i built focus i'll be honest like wincraft was different right when we had five six million users like we weren't doing interviews and getting to learn specifics it's been a really gratifying part of going from gaming to SaaS is like, with SaaS, I know a lot of the CROs, the companies that we work with, and I know what they're thinking about. And I know what they want us to be doing next.
And that's a really, really cool way to be running a company.
In terms of AI, I think there's, there's two, like learnings, which are related that I will share.
One is I think that people get really intimidated by the word AI, especially non technical folks get really intimidated by the word AI.
And I think that generative AI has made a lot of projects that used to be really sophisticated become really easy right like i think the the advent of of.
The ability to do to do what's called zero shot learning where you can just type in exactly what you want the model to do and it just does it instead of having to build a model right you can literally use english like build the model that you want and there are an enormous amount of applications for that that don't need to be a high lift to implement right i think a lot of people think of like big ai projects right like building an entire chatbot or building an entire higher intelligence system.
But what we found in Pocas is like, we can actually significantly enhance our customer experience with really, really small improvements, right?
Like one good example is within Pocas, we help you see all the data that you have across platforms.
And often across Snowflake and Salesforce and Marketo and all these different tools, you might have hundreds or even thousands of different fields.
As a sales rep, you're not going to dig through a thousand different fields, right?
So one thing that we built, and it took us literally a couple hours, was in Pocas, you can just type in what you mean, right? You can type in, I want to know if they're They're using the product, enter.
And then we'll say, all right, here's 14 different data points that'll help you answer that question.
It's an easy thing to build, right? It's like not a sophisticated use of AI.
And I think that people are often like a little afraid to do those really small sort of wins that actually go a super long way.
The other thing that I think is really important is making sure that, and it goes back to what I was talking about earlier, like making sure you're using the right tool for the job as you're working with things.
I think that like chat GPT is really on fire right now and people are really excited to build generative applications. And like we are too.
We use it all over the product.
But I think there is so, so much white space where people still haven't taken advantage of the stuff that's been out for a few years now. know.
And I think that when you're looking at different problems and jobs, like really, truly reaching for the right thing that the incredible research industry has gotten for us is important.
Andrew Miller:
Yeah, yeah, absolutely. I've already noticed a theme throughout our conversation.
And to your first point was like, don't build in a black box.
We've been talking about like, be transparent, don't throw everything in a black box between your models.
So I think that's a recurring theme that I think is really, really important here.
It's just transparency and And honestly, and talking to, I mean, that that's business 101. Talk to your customers.
I mean, you're building for an end user. You should know what that end user wants, right?
Instead of just coming from your tower and then throwing it down and say, hey, you're going to love it, right? And then it falls flat.
So I think that's a crucial, crucial point that a lot of people maybe have fear about because when you're just starting off as an entrepreneur and trying to build something, you get intimidated because you're in your mind this whole time building something that that you think they're going to love, but then you actually, you know, and you don't talk to anybody and then you release it.
And it's like, why isn't anybody loving it?
Or if you do reach out somebody to somebody, oh, are they going to not like it? You know, are they going to tear it apart?
And you take it personal as opposed to removing your own personal feelings and know that this is for a business and you're trying to build something that gets used.
So I think that's, that's a really, really good point. And I've never heard the term zero shot learning.
I'm going to use it all the time now because I really, really like it, but I see the applicability And I guess I've done that without knowing because I'm not in there writing, you know, SQL queries anymore.
I'm not like saying, hey, give me this formula for Excel or whatever thing.
I'm just saying, hey, ChatGPT, I need this to do this. Can you write the query or the formula for that for me? Thanks. Boom.
And I just paste it in. You know, it's a simple use case that doesn't require, you know, the time that it manually required in the past. And you're right.
People are intimidated because when if they haven't played around with it, they think I think it's this huge thing and it is, I mean, there's so much data and so much information that is processing, but you can start small, you know, dip your toe in it, try something that is, you know, a routine task and see how it can help you. So I love that. I love that.
Any recommendations? I mean, because you have this great background of knowledge, both from a technical standpoint and from an entrepreneurial standpoint, from a gaming standpoint.
Are there any recommendations for those interested in AI, in gaming, in entrepreneurship, like books or industry reports or podcasts or anything that you'd recommend?
Isaac Pohl-Zaretsky:
Meant yeah um in terms of entrepreneurship um i'll say like lean startup and there's a lot of books around that like there's actually the book the lean startup there's also um like six steps to epiphany books like that that's how we started focus like again there wasn't an overnight or sorry four steps of epiphany there wasn't like an overnight um revelation that we had before started like we we truly took a scientific method to doing entrepreneurship and i think those books books do a really good job describing that method and giving you really practical advice on it.
There's another book about product management called Inspired that I really like, or Inspired, that talks about how do you create tech products that people actually want to be using.
In terms of AI, honestly, a lot of my reading is a little bit more technical on that front.
One book that I love that I'd recommend folks read is called Superintelligence by Nick Bostrom.
It's basically like, what happens when AI gets so good that it's better than humans?
And it's a really interesting, like a little sci-fi, but really practical and fascinating about what does it mean for AI to sort of go to the level that we're talking about that it'll get to in 10 years.
Andrew Miller:
Awesome, awesome. We'll make sure that we put those all in the show notes so people can like directly look them up.
Looking way into the future, what would you say?
And I love to ask entrepreneurs this, you know, what's your moonshot project for Pocus, you know, for the future? Yeah.
Isaac Pohl-Zaretsky:
Like, POCUS being successful means that companies all over the world, no matter what the company looks like, can use POCUS to turn their data into revenue.
And what it means is, like, every company is using POCUS to turn data into revenue.
I think the thing that will make or break our success in doing that is, A, how can we make sure that we are bringing the right data and really good data to folks to make their jobs easier and better?
B, how can we build a platform that's really easy to use? And C, how can we really drive and demonstrate results from all the stuff that I just talked about?
So when I think about our Moonshot AI project for the future, I think about 30 or 40 different features that we're going to be building in the future. But I think the one that is most important is ultimately this question of experimentation, right?
It's the thing I was mentioning earlier with the Netflix micro examples.
It's something that we're thinking about a lot at Pocus because at the end of the day, every customer we have is asking the same question of, is my sales team spending time on the right thing?
Should we be changing our strategy in any way? And if we can build a platform that uses data, uses AI, uses all of the information, not only in your CRM, not only in your warehouse, but on the entire internet to really effectively answer that question for any type of business, like it's it's a really successful, exciting idea that we're on top of.
Andrew Miller:
Yeah, absolutely. Absolutely. I love it. So if our listeners want to follow you, what are the best places to, you know, check you out?
Isaac Pohl-Zaretsky:
Yeah. Being in in Pocus land, I spent a lot of time thinking about Pocus.
So really encourage folks to go to our website, Pocus.com.
If any of this sort of data to revenue type conversation is interesting to you, please fill out your email and we'd love to have a chat.
We also have an awesome newsletter where we talk with tons of experts at the top companies in B2B SaaS about how they're working on turning their data into revenue.
Encourage you to sign up for that, as well as our community.
We have an amazing community of many thousands of people in Slack who are thinking about these same challenges of how do I turn my data into revenue?
We are always looking for new members to come and join us.
For me personally, I'm mostly a LinkedIn guy. It's Isaac Polzarewski on LinkedIn, and I'll always love to connect with you.
Andrew Miller:
Perfect, perfect. Well, I'll put again, I'll put all those links into the show notes here so that everybody can check it out.
My final question is, do you have any words of wisdom or final thoughts that you'd like to leave with the audience before we close out?
Isaac Pohl-Zaretsky:
I think that it is really, really exciting what has happened in the last couple of months and years.
And I think it's like really, really exciting about what's about to happen.
And I think it's really easy to look at all the changes that have happened in our world and take a really pessimistic approach, right, of the world's going to end or my job's about to disappear, or these AI overlords are going to take over.
And like, to be fair, I think that's a path, right? I think it's really important to be ethical and really, really thoughtful and careful with the approaches we're taking.
And I think there are cases where folks are moving too quickly or not being thoughtful with the ways they're using these new technologies.
But I also think that historically, the fact that technology makes our lives better is a trend that I expect to continue.
And like, as I look at the changes of what's happening in my industry and every other industry, as AI has taken over.
It's a path forward that I'm really excited about. And I think being cautious around what we are doing and being pessimistic around what could go wrong is important.
But I also think holding that optimism in mind of like we could truly change how people's lives are spent is really, really exciting.
Andrew Miller:
Nice, nice. Well, thank you so much for your time, Isaac.
This has been a pleasure. I have literally like pages and pages of show notes here that I'm going to be writing up after this, but a true pleasure to have you on the show, man. Really appreciate it.
Isaac Pohl-Zaretsky:
Absolutely. Thanks very much, Andrew. I appreciate it.
Andrew Miller:
All right. Bye-bye.
Recent Podcasts
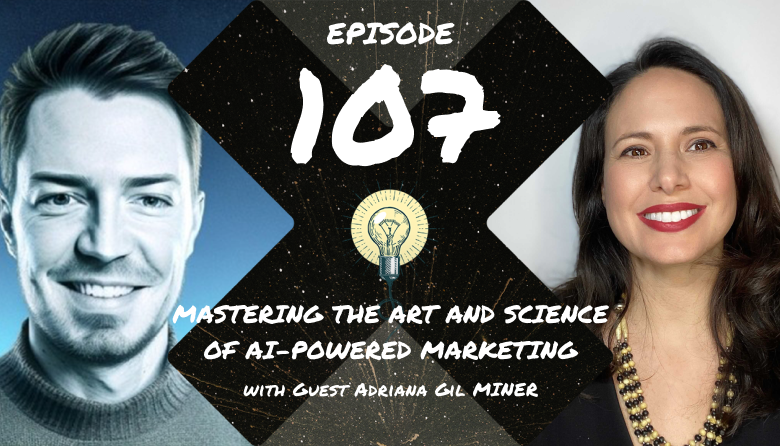

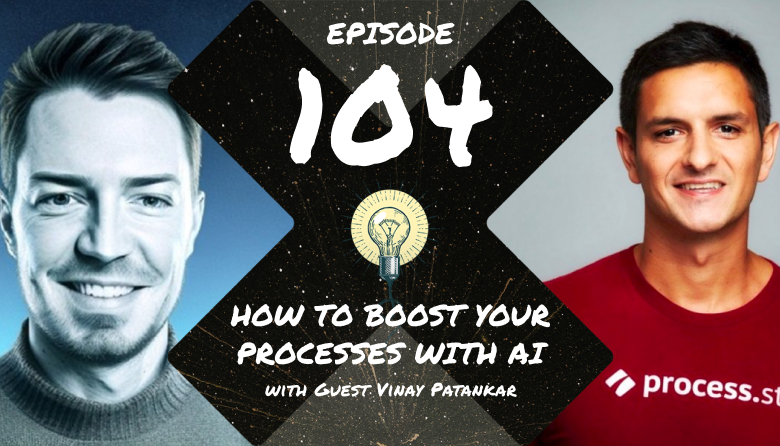